It all starts here
The RV Advantage
This is a place where you can unlock substantial exposure, gain experience, and even make entire career shift – all within one organization.
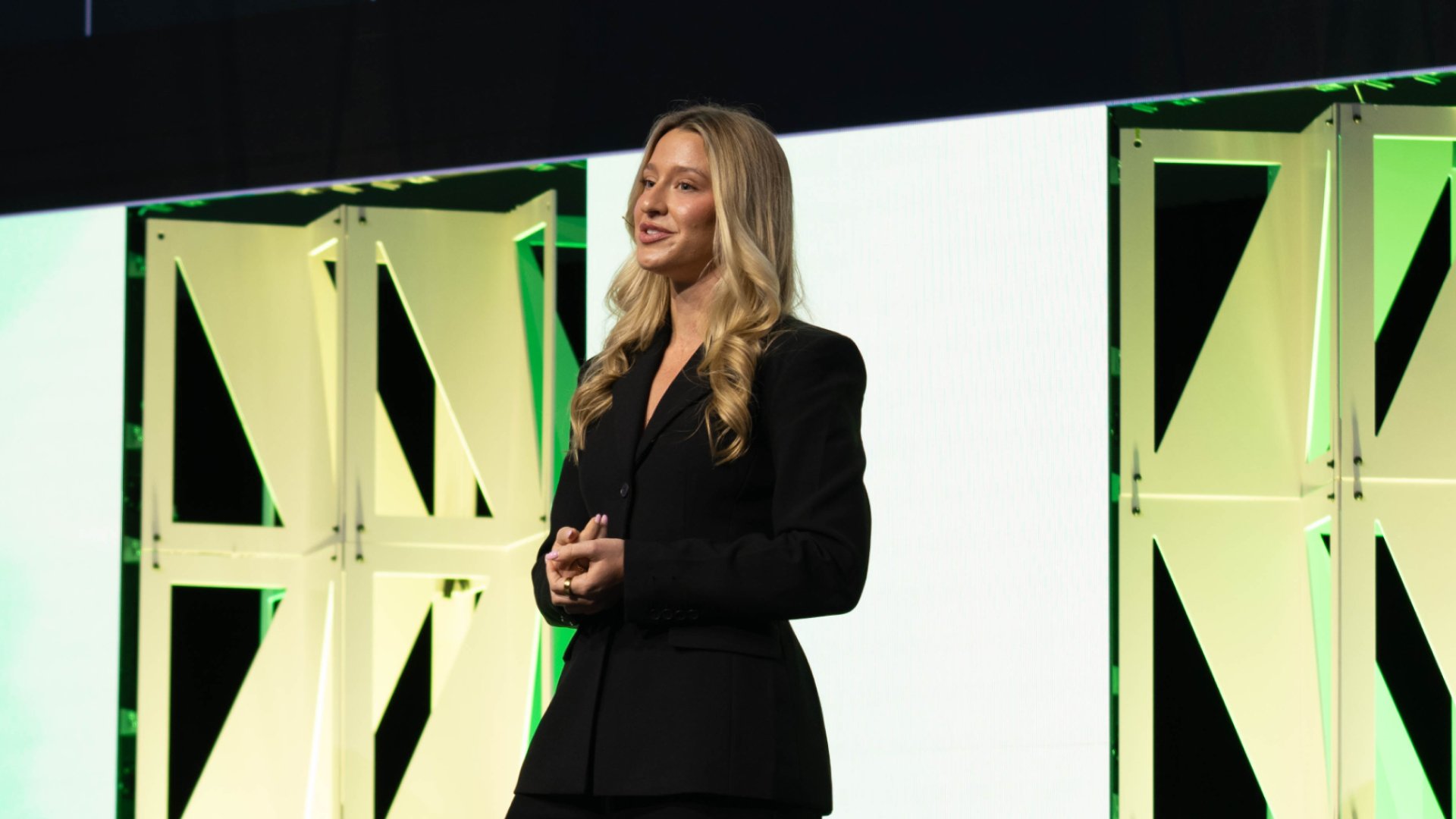
Meta
Fast-track your career
RV is the place you come to kick-start, boost, or reignite your career.
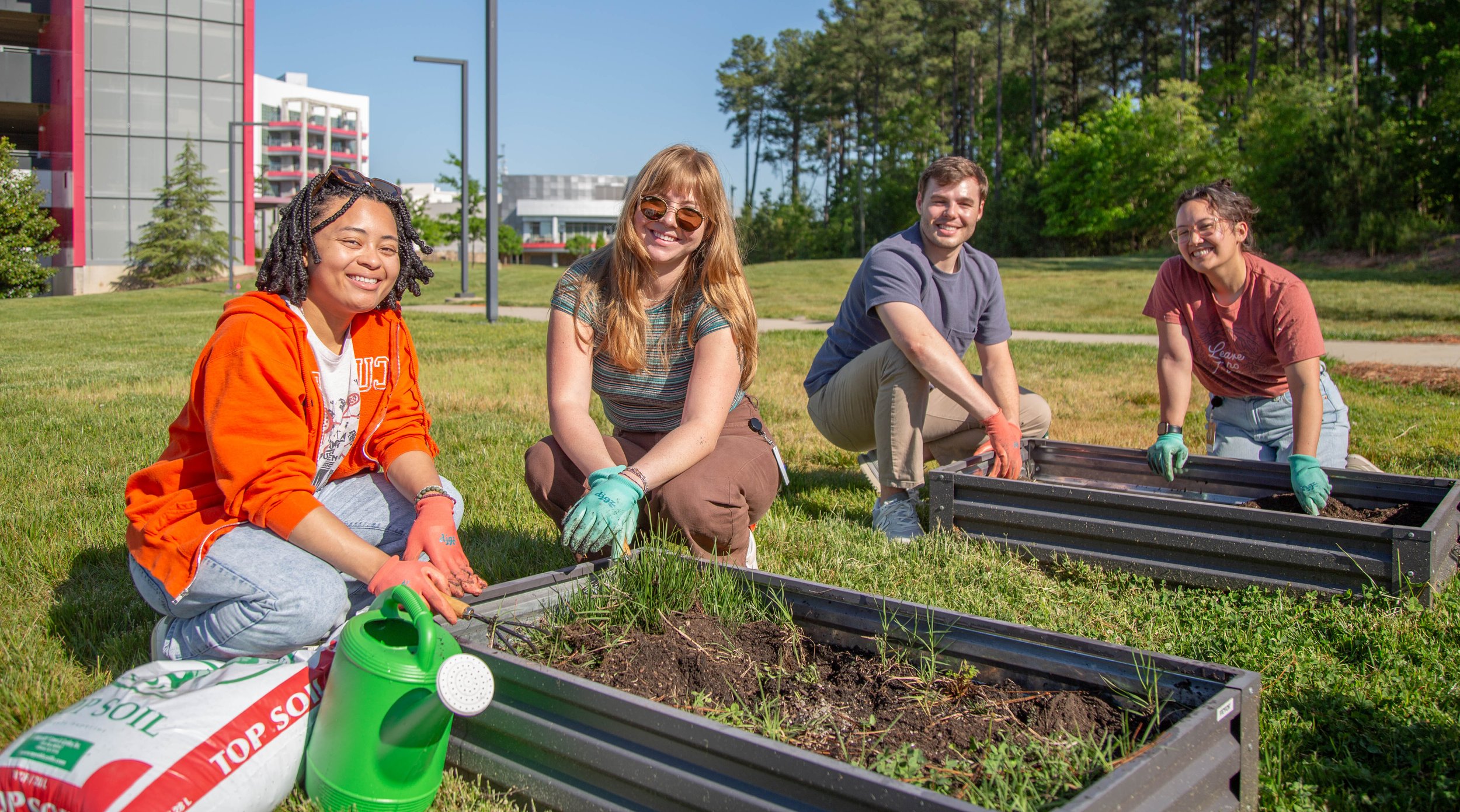
Meta
Make it your own
When it comes to our work and your career, there are no dead ends. If it doesn’t exist we’ll build it. If it’s not working, we’ll change it. We think it’s fun to do bold things, fast.
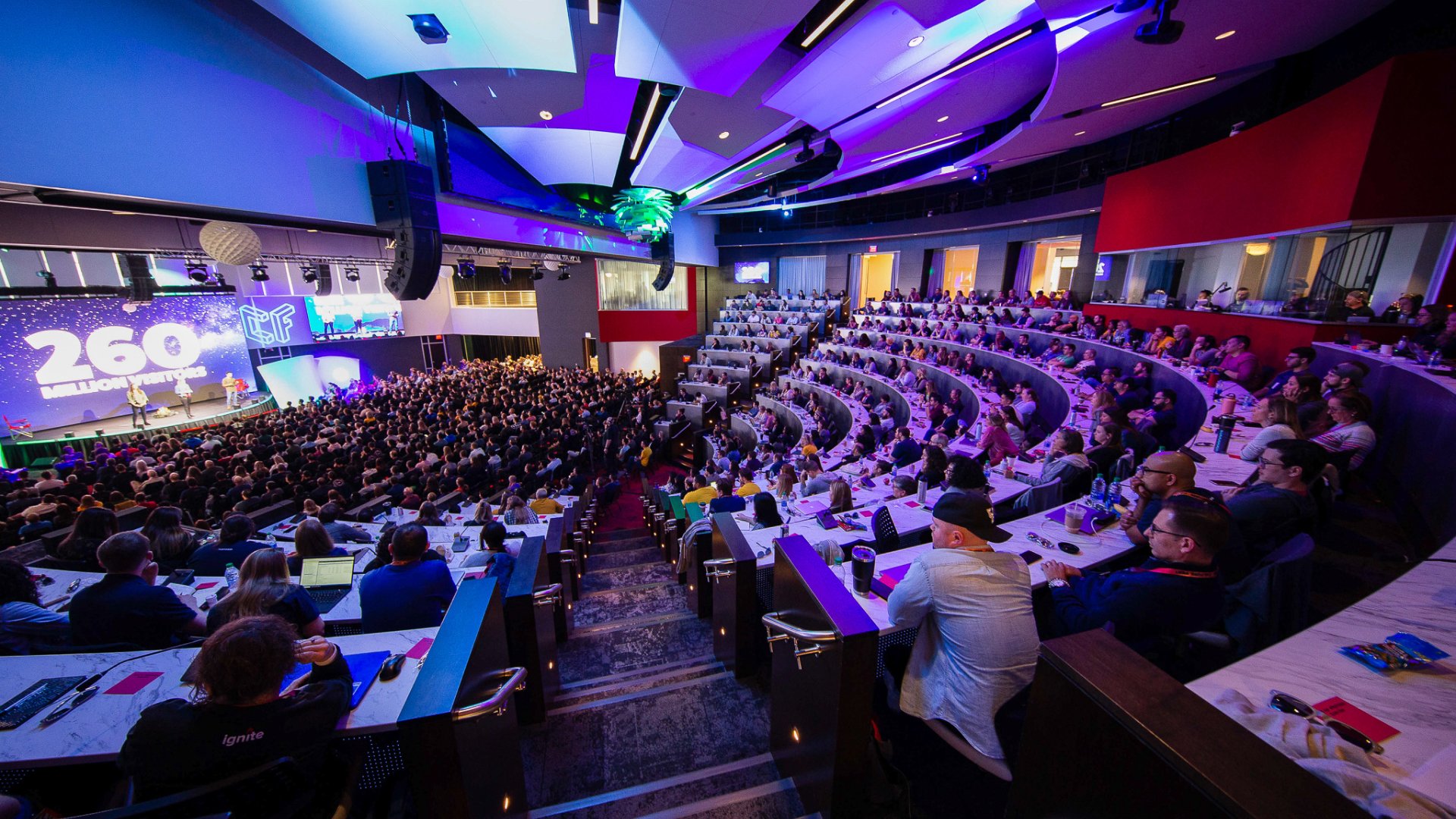
Meta
Be part of something big
Red Ventures is many teams and many business models, but one culture, one community. Get the best of both worlds – a startup mentality with the stability of global company.
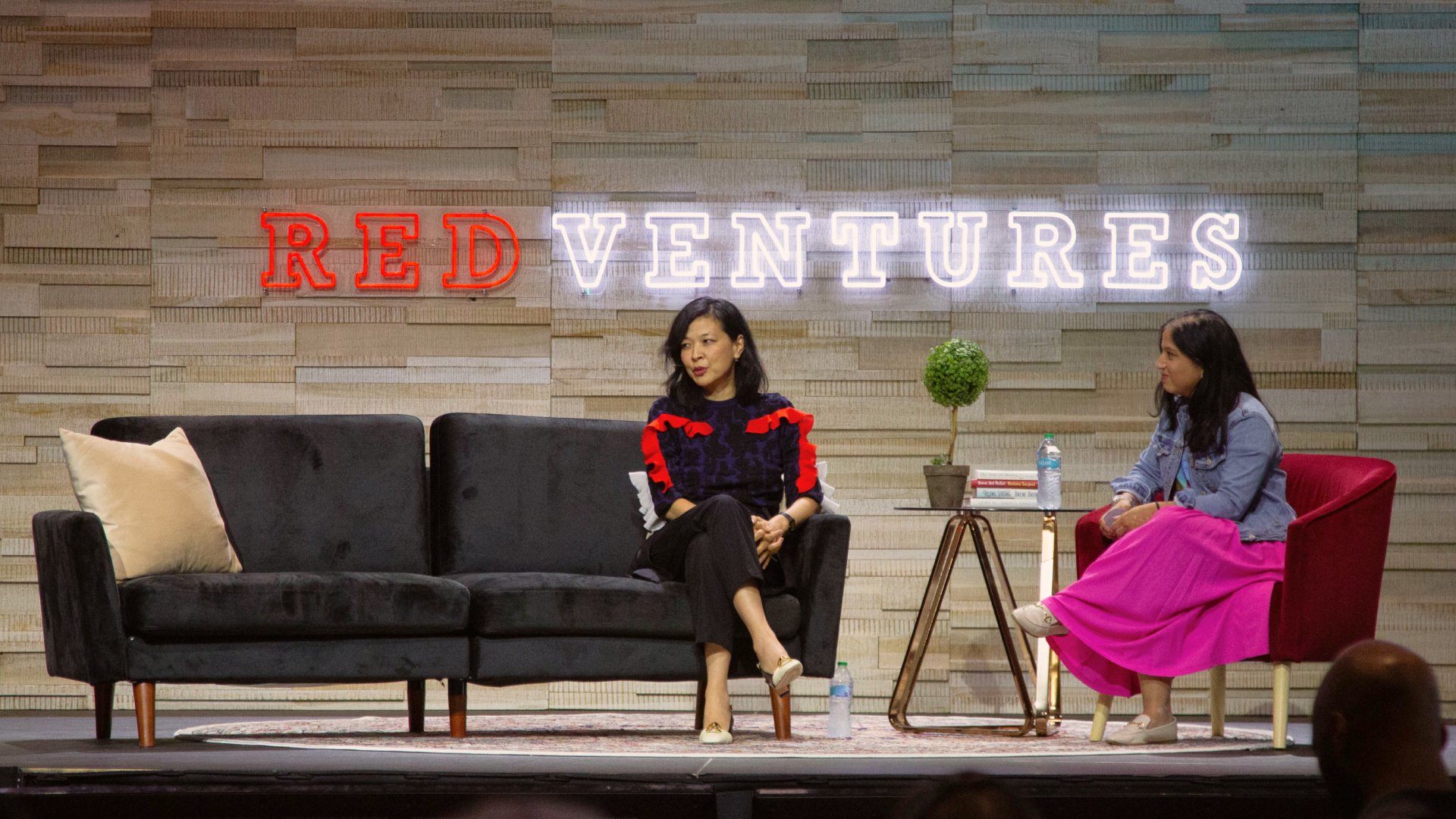
Meta
Find outside inspiration
Take advantage of unmatched access to world-renowned entrepreneurs, athletes, and guest speakers via our RedTalks series and HQ events.
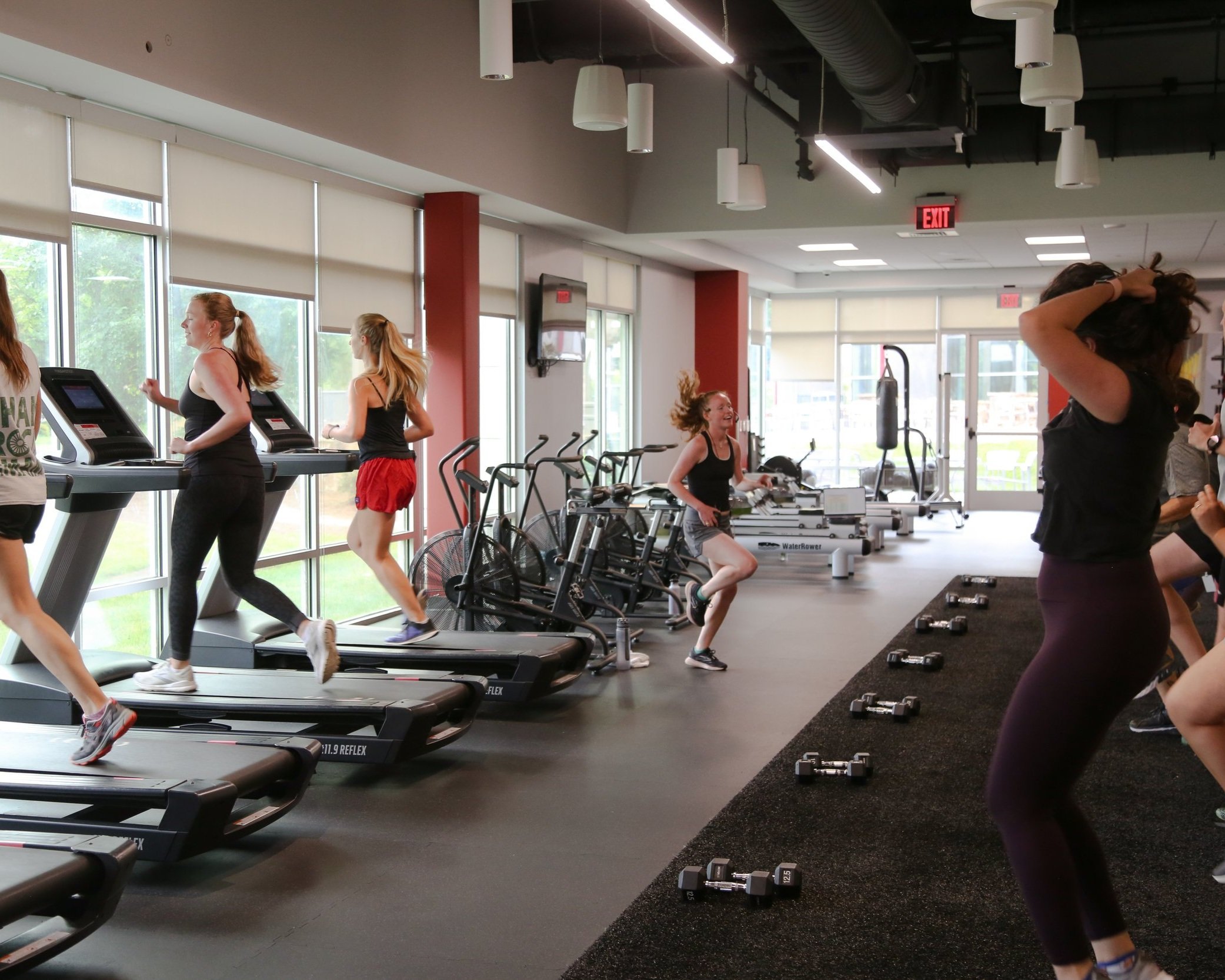
Meta
Enjoy world-class perks
Red Ventures HQ is a “see it to believe it” campus, and our inclusive benefits include generous health & wellness plans, PTO, parental leave, mental health benefits, and more.
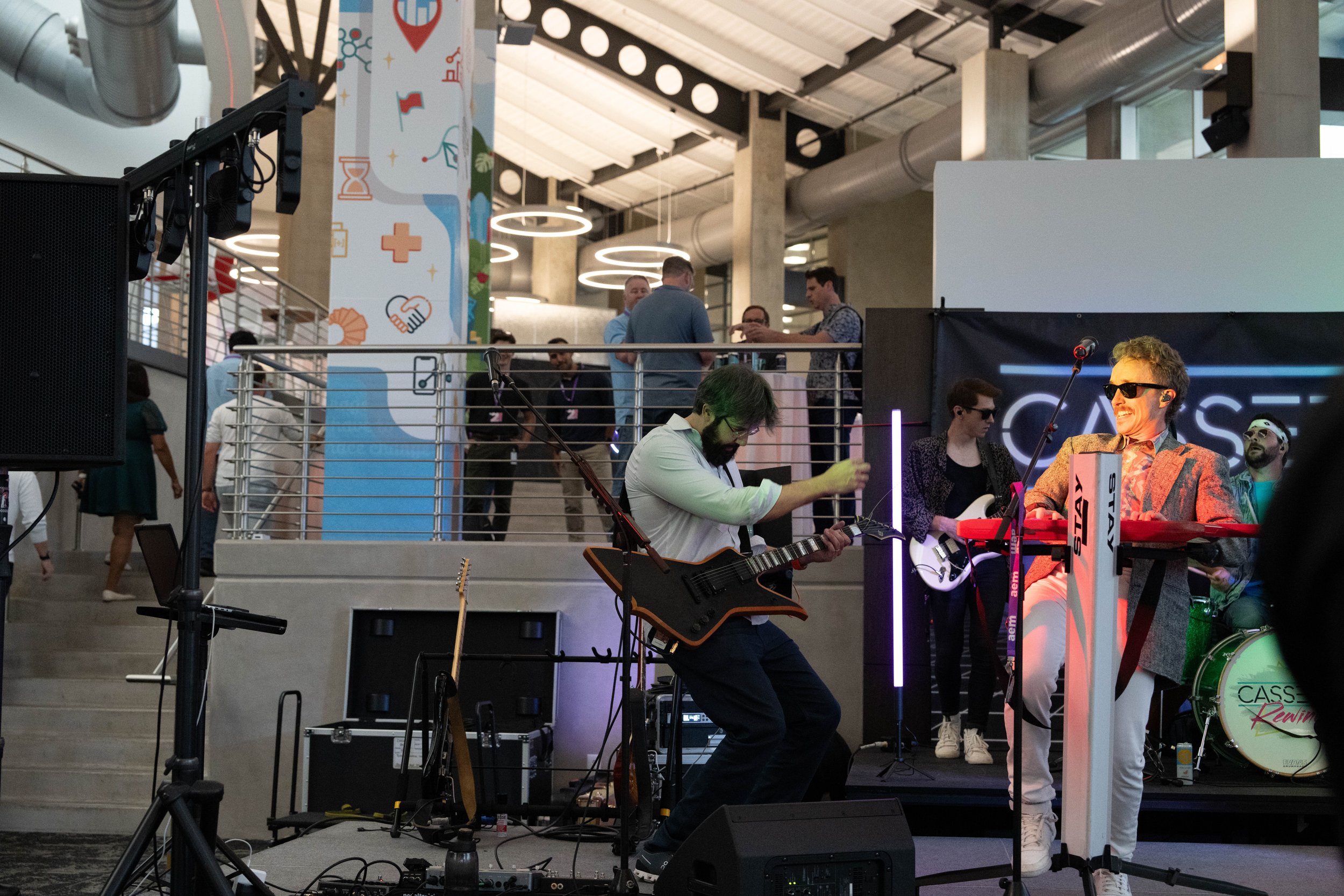
Meta
Have fun (seriously)
What other company has their own inflatable noodle-man, puts senior leaders on stage to rock out guitar solos, and takes silliness as seriously
we do?
we do?
A sense of mutual respect and mindfulness permeates our culture-in fact, it’s the key to our success.
Employee Benefits
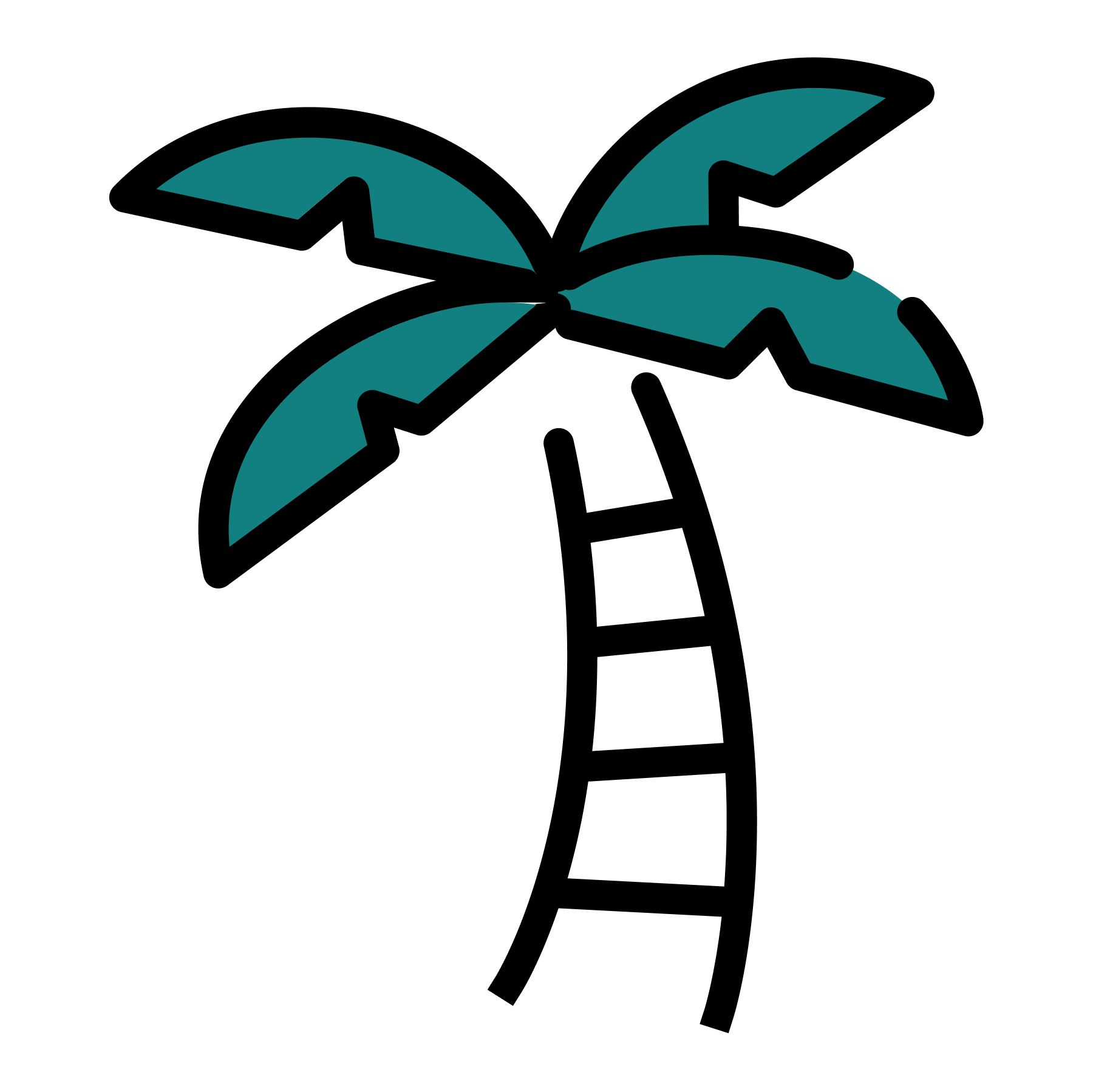
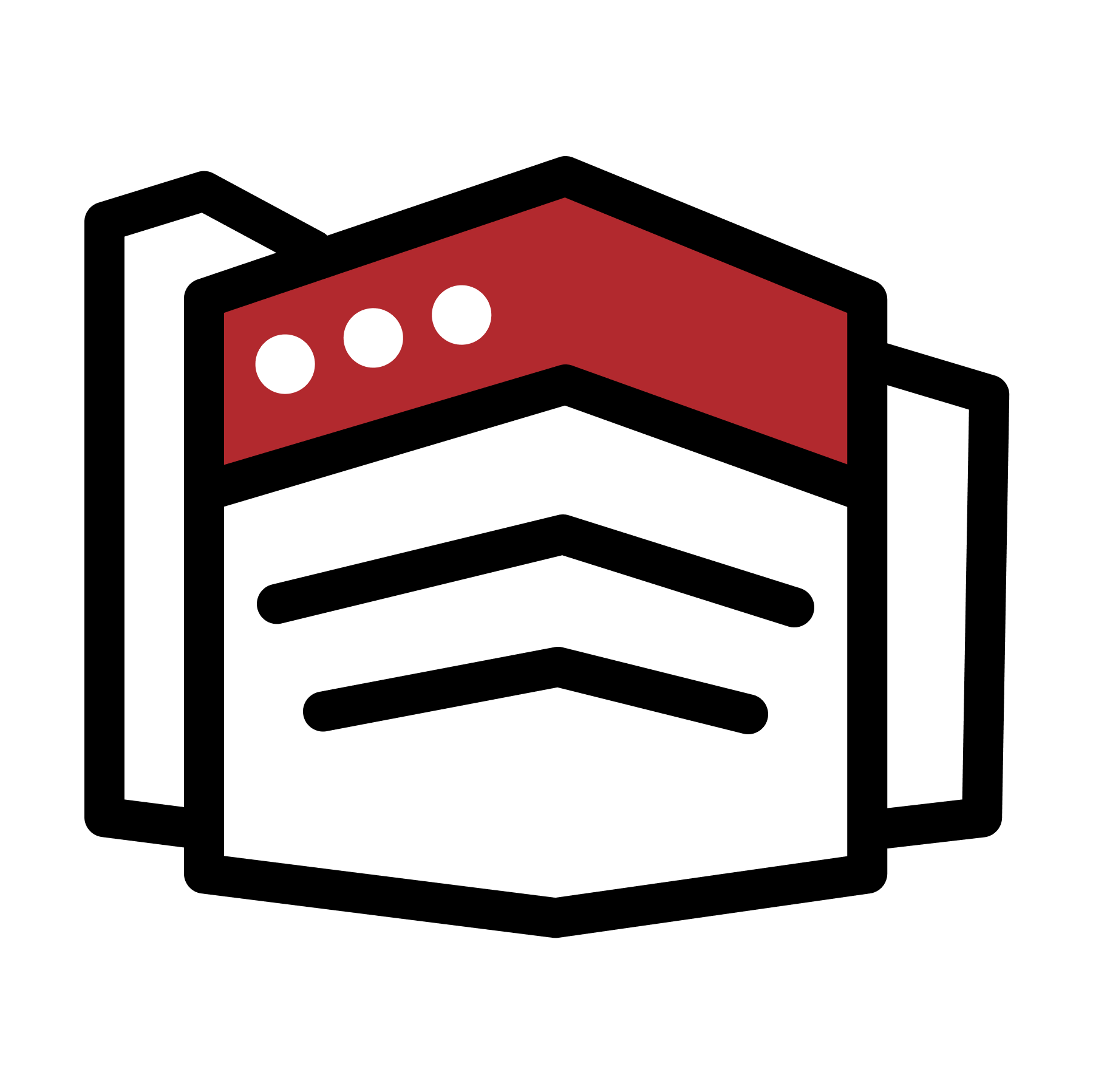
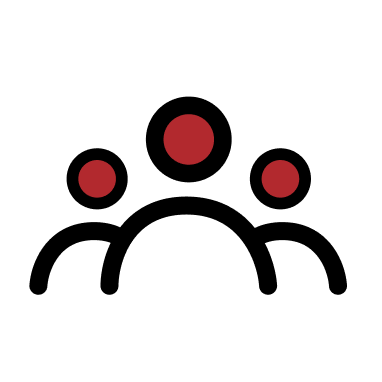
A sense of mutual respect and mindfulness permeates our culture-in fact, it’s the key to our success.
The Phenom Market Life
A career at Phenom Foods Market is more than the work you do. impact on the community, your personal growth and team members.
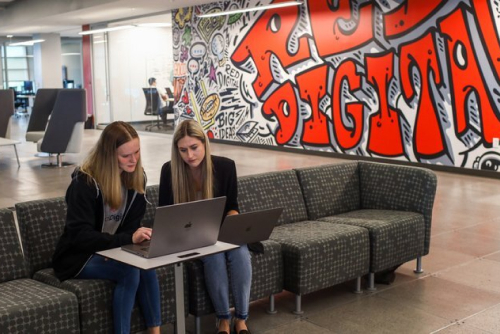
lorem ipsum
Growth Marketing & Business
We do more than pull numbers and organize spreadsheets. At RV, you’ll develop a deep understanding of consumer behavior in order to dramatically improve digital experiences.
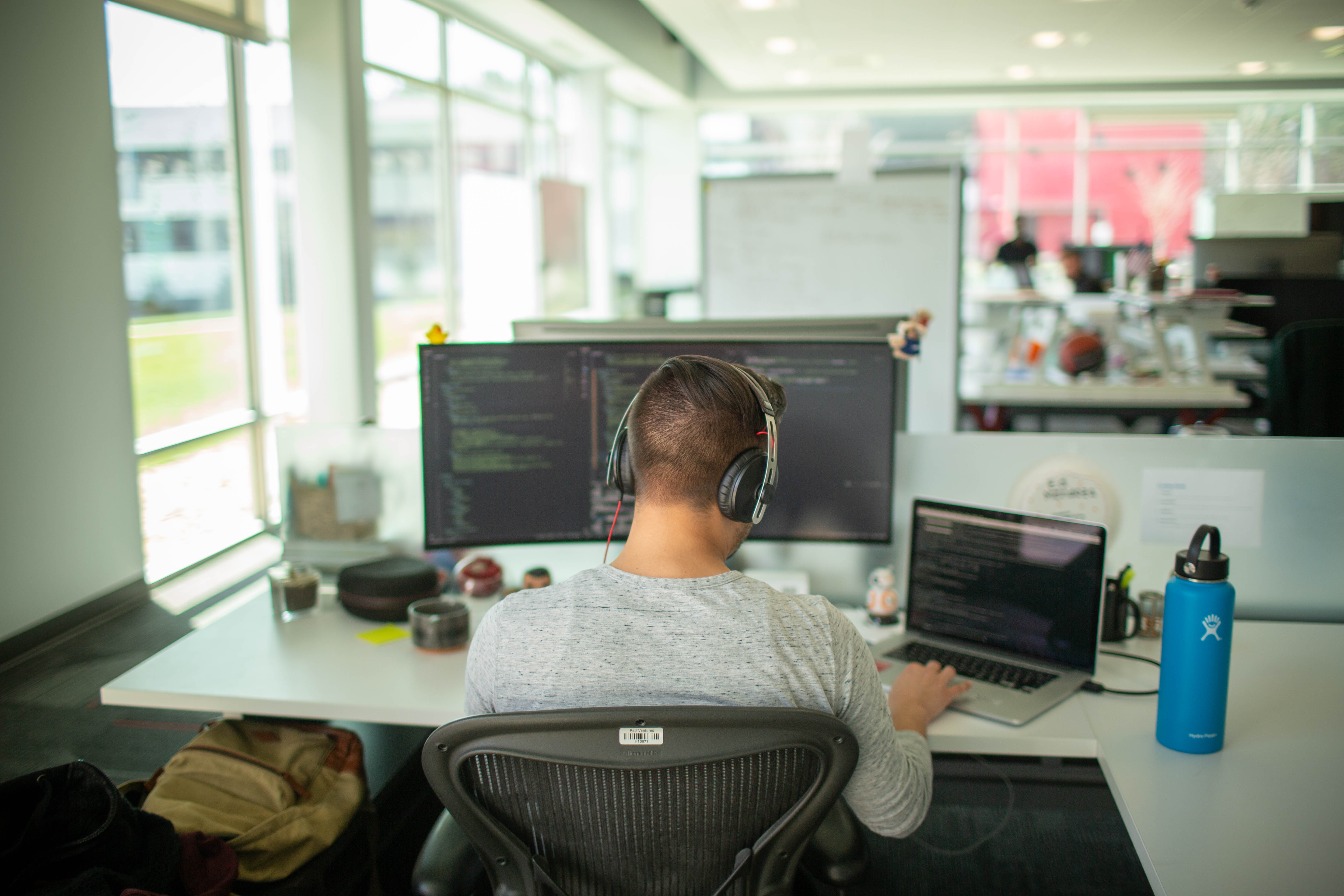
lorem ipsum
Tech, Data & AI
Looking for a chance to work on an award-winning team, help others ask better questions, and make data-based decisions? This is the place for you.
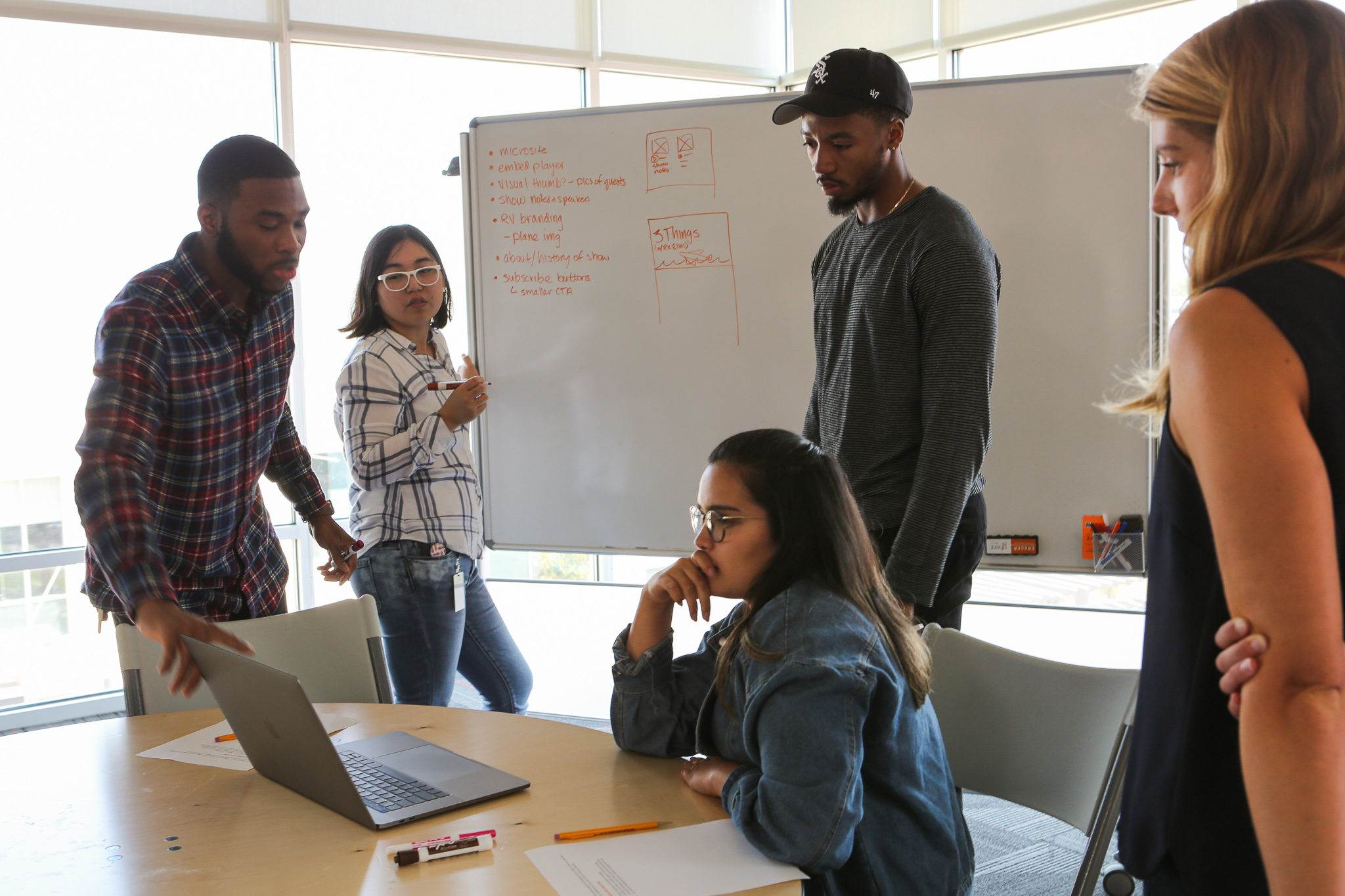
lorem ipsum
Creative & Editorial
As a Creative at Red Ventures, you’ll develop premium content and intuitive, seamless experiences that help millions of people make life’s biggest decisions.
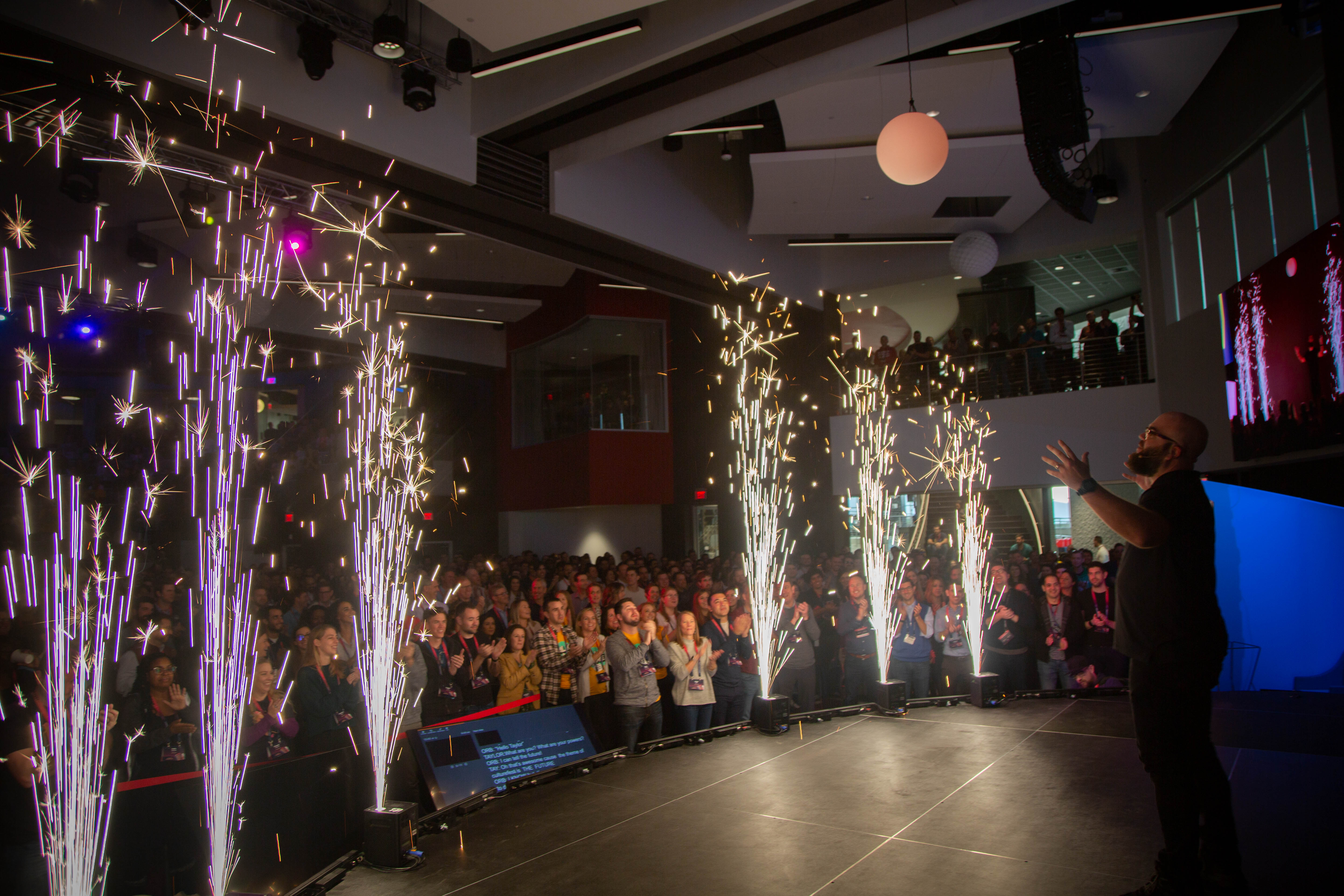
lorem ipsum
Corporate Support
We believe in empowering our employees to be the authors of who we will be. It takes a unique support team working hard behind the scenes to make that a reality.
A sense of mutual respect and mindfulness permeates our culture-in fact, it’s the key to our success.
Sign up for Job Alerts!
Lorem ipsum dolor sit amet consectetur adipisicing elit. Error voluptate architecto eaque, minus quis delectus adipisci.
Our Commitment
Our goal is to listen, engage, and activate voices across Red Ventures to ensure that all identity groups and perspectives are represented in our work. Our aspiration is to have diverse teams at every level of the company, and we seek candidates who bring unique personal experiences, curiosity and an eagerness to embrace diversity, equity, and inclusion.